Improving empiric antibiotic prescribing by applying a Bayesian decision theory approach to phenotypic and genomic resistance data
Using surveillance data for phenotypic and genotypic resistance to inform appropriate antibiotic prescribing
What is the problem?
Antimicrobial resistance is in part caused by the inappropriate prescribing of antibiotics. Since the publication of Lord Jim O’Neill’s AMR Review in 2014-16, there have been inroads to reduce the use of antibiotic prescribing through antimicrobial stewardship initiatives. However, the majority of antibiotics administered are prescribed empirically for presumed bacterial infection without any testing which can lead to the overuse and inappropriate use of antibiotics.
Sepsis is a life-threatening consequence of bacterial bloodstream infection. It requires rapid antibiotic therapy, and in almost all cases, the antibiotic susceptibility profile of the bacterium causing the infection is not known. Hence, working “in the dark”, prescribers must balance the use of antibiotics where rates of resistance are low – meaning treatment success is more likely – but also be mindful of unnecessarily using the latest antibiotics, because that will inevitably drive resistance. Such empiric antibiotic choice can therefore lead to treatment failure, or unnecessary antibiotic use. If treatment fails, then the clinician must decide which antibiotic to try next, often without any additional information.
A possible solution?
Most initial empiric antibiotic choices for bloodstream as well as other infections are informed by an understanding of local antibiotic resistance patterns from isolates recently microbiologically investigated. While this local antibiotic resistance data is widely available, particularly for urinary tract and bloodstream infections it is currently an underused resource due to a lack of understanding of circulating resistance mechanisms, the interplay between mechanisms and how this data can be used to guide prescribing.
The aim of this project is to improve empiric antibiotic prescribing, by collecting antibiotic resistance data collected for the Bristol, North Somerset and South Gloucestershire Integrated Care Board. The team will then build this data into a Bayesian decision making tool, integrating not just how common resistance to drug X is but how common resistance to drug X and drug Y occur together in different population groups. The aim is to choose antibiotics more wisely, to reduce the incidence of treatment failure and the emergence of resistance.
Outcome and next steps
The team has completed data collection for Gram negative bloodstream infections and developed a Bayesian model that represents trends in resistance and co-resistance in different patient groups that overcomes barriers seen when small numbers of infections are seen in a particular group. Factors that group patients include age, sex, underlying health condition and hospital location, e.g. ICU. The model and its findings have been accepted for publication and preprinted (Bamber et al., 2023 MedRxiv doi.org/10.1101/2023.11.03.23298025). Future work involves rolling this work out nationally, alongside UKHSA colleagues, and applying it to data from urinary tract infections in primary care, helping to establish the best first line antibiotic.
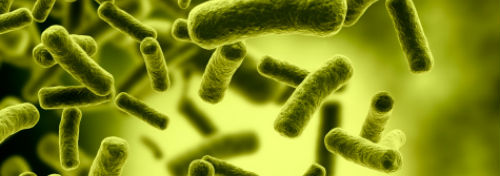
Researchers involved
- Dr Philip Williams (University Hospitals Bristol and Weston NHS Foundation Trust)
- Prof Andrew Dowsey (Dept of Population Health Sciences, Bristol Medical School and Bristol Veterinary School)
- Prof Matthew Avison (School of Cellular and Molecular Medicine)
-
Dr Ranjeet Bamber (Dept of Population Health Sciences)
-
Dr Brian Sullivan (Dept of Population Health Sciences)
-
Dr Leo Gorman (Jean Golding Institute)
External collaborators
- UKHSA
- Severn Pathology
Funding
- Medical Research Council Clinical Academic Research Partnership (MRC CARP award)
- Medical Research Foundation
- HDR-UK
Dr Philip Williams
email: pw5083@bristol.ac.uk