Statistical Methods for Causal Inference
This programme aims to develop statistical toolkits and methods which can be used to improve the understanding of factors that cause different health conditions. This is carried out under five workstreams:
1: Develop Methods to model heterogeneity and causal effects
Methods to help understand how people differ in their response to treatment: People may respond differently to treatments - for some they may be effective, but for others less so. This might be due to differences between people which remain constant across their lives, or differences that change across lifespan (e.g. treatments may be more effective at certain ages). We are creating statistical methods to model such diversity in the relationship between causes and health outcomes.
2: Develop methods to model measurement error and its impact on causal analyses
Identifying unreliability in health measures, and correcting their impact on study conclusions: Health conditions, and their possible causes, may be measured unreliably (error) which can lead to the wrong conclusions (bias) when trying to describe the relationship between them. We are developing methods to help identify how unreliable measures are, and to try to correct bias resulting from such measurement to enable more sound conclusions to be drawn from studies.
3. Develop methods to address complex causal and lifecourse hypotheses
Understanding the complexity of the causes of health outcomes: We are creating a range of methods to better describe the complex range of causes of health outcomes that can arise during a person’s lifetimes. This will allow us to better understand the likelihood of getting certain conditions.
4. Develop methods for detecting and mitigating selection bias
Detecting and mitigating bias in selecting participant data: Not everyone who is eligible to participate in a health study does so, and sometimes people with certain characteristics are more likely to participate than others. The extent to which different types of people “drop out” of studies may vary. This non-random tendency to participate can cause “selection bias”. For example, an apparent association between age and COVID-19 may reflect the types of people who tend to be users of an app (young people) which collects such measures, rather than reflecting a true underlying association. We are developing ways to detect and minimise selection bias.
5. Triangulation methods to quantitatively combine MR studies and RCTs
Combining results from different approaches to understanding causes of health outcomes: Triangulation brings together results from different approaches and study designs, particularly where these have different sources of bias. We are developing methods to integrate the results from two different study designs, to better understand the causes of health outcomes, by drawing on the strengths and acknowledging and addressing the weaknesses of each approach. These are Mendelian Randomization studies, which aim to understand disease causes and treatments through genetics, and randomised controlled trials (RCTs), which aim to understand disease causes and treatments by randomly assigning people to different interventions, e.g. a test drug and a placebo.
Research highlights
Instrumental variables (IV)
We developed a new F-test statistic for determining whether the IV estimator in linear models suffers from weak instrument problems where there are multiple treatments that are potentially confounded. The problem here is that the instruments have to predict the multiple treatments jointly. The new conditional F-test statistic is shown to have similar properties to the standard F-test for the one-treatment model, and standard weak-instrument critical values can be used. It has been included in the user written software ivreg2 in Stata.
Key publication: Sanderson, E, Windmeijer F (2015). A Weak Instrument F-test in Linear IV Models with Multiple Endogenous Variables. Journal of Econometrics. Epub ahead of print:
Modelling change over the lifecourse
We developed a new method to identify which of a small set of hypothesized models explains most of the observed outcome variation, and showed that our approach identified the correct model with high probability in moderately sized samples, but with lower probability for hypotheses involving highly correlated exposures. Identifying a single, simple hypothesis that represents the specified knowledge of the life course association allows more precise definition of the causal effect of interest.
Key publication: Smith AD, Heron J, Mishra G, Gilthorpe MS, Ben-Shlomo Y, Tilling K (2015).
Model Selection of the Effect of Binary Exposures over the Life Course. Epidemiology. Epub ahead of print: PMID: 26172863
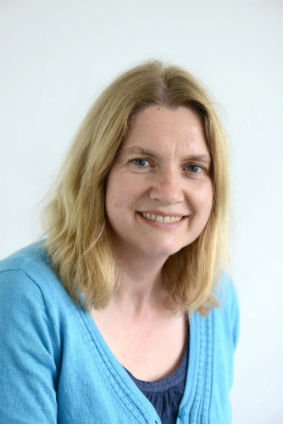