Data mining epidemiological relationships: integration of causal analysis with published evidence
Programme overview
Population health research is being transformed by the increasing wealth of complex data. New high-dimensional epidemiological datasets provide novel opportunities for systematic approaches to understanding the relationships between risk factors and disease outcomes. This programme is building on our successes in collating data (e.g. IEU OpenGWAS) and implementing software to automate causal inference using Mendelian randomization (e.g. MR-Base), and in literature mining (e.g. MELODI). We have implemented a new graph database (EpiGraphDB) that integrates causal estimates with comprehensive data on relationships between traits, risk factors, biomarkers, intervention targets and diseases. Using EpiGraphDB we are developing new methods to explore the relationships between risk factors and disease, enabling new causal hypotheses to be generated and explored.
Aims and objectives
We aim to:
- Systematically integrate biological contextual information with causal estimates generated using Mendelian randomization
- Develop novel approaches to identifying, validating and prioritising potential causal estimates in the context of a wide array of other information
- Utilise our database to inform the development of new Mendelian randomization methods that address pleiotropy
- Apply the data and approaches from (1) to (3) to investigate the causal risk factors in cardiovascular disease and cancer.
Research highlights
See group website for recent updates.
MR-Base and IEU OpenGWAS
MR-Base (www.mrbase.org) is an openly accessible R (R statistical language) package, a web application and a comprehensive database of GWAS studies (the MRC-IEU GWAS database) including 3.4 billion genotype/phenotype association results from 974 GWAS studies published by 36 consortia. MR-Base enables automation of two-sample MR using 11 MR methods (including MRC-IEU methods addressing pleiotropy).
2020 update:
- Database deployed on Oracle Cloud Infrastructure as part of an ongoing collaboration with Oracle
- Full summary statistics made available in a new VCF format developed by us: Lyon et al, bioRxiv 2020
- Pre-print of the IEU OpenGWAS platform published, the core data resource underpinning MR-Base: Elsworth et al, bioRxiv 2020
Drug target prioritization
We are working with various pharmaceutical companies on approaches to prioritise drug targets using available data. Our initial work has focused on the use of Mendelian randomization and genetic colocalization, but this is being extended to use other data within EpiGraphDB to triangulate the evidence for potential targets and use molecular pathway data to gain better insights into how these function.
2020 update:
- Use of MR and colocalization to prioritise potential drug targets, with results in EpiGraphDB: Zheng et al, Nature Genetics 2020. Also see our press release and animation.
MELODI
MELODI (www.melodi.biocompute.org.uk) mines the biomedical literature for mechanistic relationships between epidemiological concepts using a graph database (Neo4J, neo4j.com) that incorporates concepts (phenotypes, diseases, etc) from MeSH (Medical Subject Headings) and the SemMedDB Database extracted using the SemRep text-mining software.
2020 update:
- Release of MELODI-Presto for identifying potential molecular intermediates using literature mining: Elsworth et al, Bioinformatics 2020
LD Hub
LD Hub (ldsc.broadinstitute.org) - In collaboration with Ben Neale (Broad Institute) and Dave Evans (University of Queensland) we developed LD Hub, a web application that performs linkage disequilibrium (LD) score regression. This enables us to automatically estimate the genetic correlations between a wide range of traits and diseases.
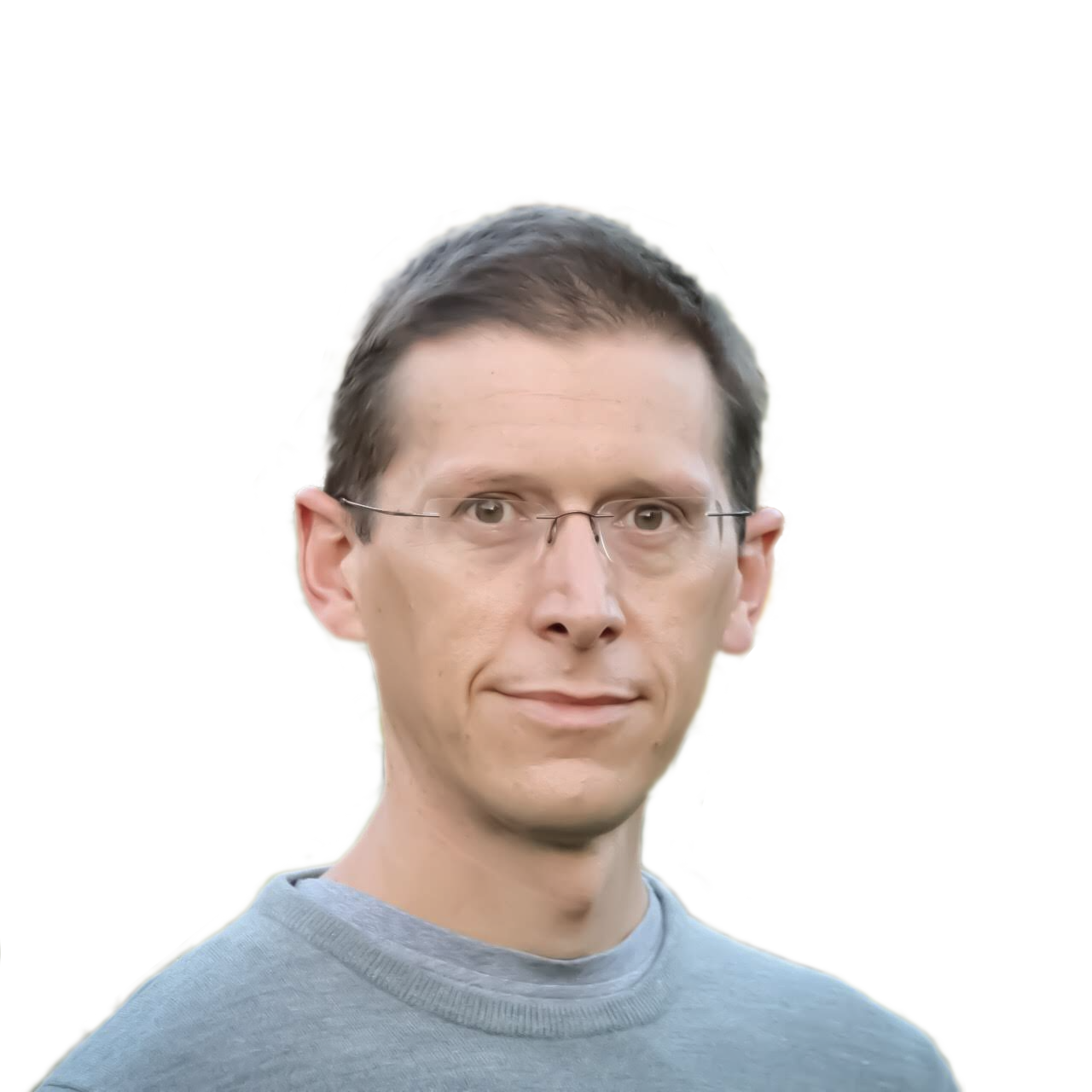