Current Year
This page contains information on Seminars that occurred during the 2024-2025 Academic Year. Details of upcoming talks, and how to attend, can be found on the main page: Seminar Series
28th May 2025, Why did you do that? Use actual causality to explain the problems (or the lack of problems) in your life
In this talk I will survey the existing and suggested connections between the theory of actual causality and a number of applied areas in CS, focusing on explainability of black-box systems, such as neural networks.
Prof. Hanah Chockler
Professor Hana Chockler is a Professor in the Department of Informatics, King’s College London. In 2021-22, in parallel with her faculty appointment, she has been working as a Principal Scientist in a start-up company causaLens, whose mission is to introduce causal reasoning to support human decision-making in a variety of domains. Prior to joining KCL in 2013, Prof Chockler worked at IBM Research in the formal verification and software engineering departments. Her research interests span a wide variety of topics, including formal verification and synthesis, causal reasoning and its applications, and, most recently, computational questions related to the regulation of AI systems. Prof Chockler is a co-lead in the CHAI Hub (Causality in Healthcare AI systems), working on explainability of AI systems in healthcare.
21st May 2025, AI Security: Language Models, Data Encryption, Software Verification
Neural networks are slowly getting integrated into safety-critical systems. Unfortunately, we still lack a full suite of algorithms and tools to guarantee their safety. In this talk, I will present a few open challenges in AI safety and security: consistent behaviour in language models, machine learning over encrypted data, model compression with error guarantees, bug-free floating-point software. Here, I will claim that formal methods are the key to address these challenges, as long as we can settle on an unambiguous specification.
Dr. Edoardo Maino
Dr. Edoardo Manino is a Lecturer (Assistant Professor) in AI Security at The University of Manchester. He has a lifelong interest in AI algorithms, from symbolic AI to machine learning. He spent most of his research career at Russell Group institutions in the UK, funded by EPSRC and the Alan Turing Institute. His background is in Bayesian machine learning, a topic he was awarded a PhD from the University of Southampton in 2020. In the past years, he has been interested in all variations of provably safe machine learning, from pen and paper proofs on tractable models to automated testing and verification of deep neural networks and large language models. He has a strong record of cross-disciplinary publications, spanning human computation, software engineering, hardware design, signal processing, network science and game theory.
26th March 2025, Multi-scale Feedback Systems: a Design Pattern for Large Complex Adaptive Systems
Abstract:
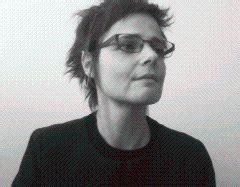
19th March 2025, Assuring Safety in the Face of the Unpredictable
Venturing into the world of autonomous systems, this talk explores the intricacies and challenges of assuring safety in a realm where, as 19th-century philosopher William James put it, “the world is a blooming, buzzing confusion.” The spotlight is on learning-enabled systems, a domain facing urgent safety challenges in our rapidly advancing technological landscape. The presentation revisits the concept of resilience, opening up a vital discussion on the necessity of safety in the face of unpredictability. It lays out the current challenges with a keen eye on the complex balance between system utility and safety. Potential solutions are proposed, providing thought-provoking insights into how we can enable these systems to adapt themselves to diverse contexts without compromising their safety. This talk takes you on a journey into the heart of self-adapting, resilient systems, exploring their complexities, their potential, and their critical role in our future. It's a riveting exploration of a new generation of systems that continuously adapt to meet the unpredictable challenges of the world around them. Based on pertinent examples and current research, this talk not only delves into the dynamics of learning-enabled systems and their safety assurance but also underscores the challenges that remain to be addressed, thereby shedding light on these systems' promising future potential.
Prof. Mario Trapp
Prof. Mario Trapp is Executive Director of the Fraunhofer Institute for Cognitive Systems IKS. In 2005, he obtained his PhD from TU Kaiserslautern, where he also did his habilitation in 2016. He also joined Fraunhofer IESE in 2005, where he started off as a head of department in safety-critical software before becoming head of the Embedded Systems division from 2009 to 2017. After being appointed Acting Executive Director of Fraunhofer ESK (now Fraunhofer IKS) in Munich on January 1, 2018, he assumed this role on a permanent basis on May 1, 2019. In addition to this, Mario Trapp has been a Full Professor at the Technical University of Munich (TUM) since June 1, 2022. He is a Full Professor for Engineering Resilient Cognitive Systems at the School of Computation, Information and Technology CIT. Prior to this, he was an Adjunct Professor in the Department of Computer Science at the TU Kaiserslautern. For many years, Mario Trapp has been contributing his expertise to the development of innovative embedded systems in the context of successful partner projects, in cooperation with both leading international corporations and small and medium-sized enterprises. Currently, his personal research focuses on safety assurance and resilience for cognitive systems, which form the technological basis of many future scenarios such as Industrie 4.0 and automated driving. Mario Trapp has authored numerous international scientific publications. He is also a member of the Bavarian State Government’s Council on AI (Bayerischer KI-Rat) and the Bavarian State Ministry of Economic Affairs, Regional Development and Energy’s AI — Data Science (KI — Data Science) expert panel. Moreover, he is the chair of the EWICS association.
12th March 2025, Dr. Mohammud Junaid Bocus, Mr Senhui Qiu
Towards Green IoT: Pioneering Sustainable IoT with Hybrid Optical-Radio Communication and Printed Electronics
Achieving sustainability and optimal resource usage in Internet of Things (IoT) technologies requires informed decision-making during design and implementation. The EU-funded SUPERIOT project develops a truly sustainable and highly adaptable IoT system by leveraging a dual-mode communication approach that integrates optical and radio technologies. The use of printed electronics in SUPERIOT aims to enable the creation of eco-friendly IoT nodes capable of seamlessly switching between optical, radio, or hybrid connectivity. This hybrid communication system maximizes the strengths of both wireless methods, enhancing flexibility and efficiency. To further minimize environmental impact, the energy-autonomous nodes are designed to harvest energy from optical and radio sources while supporting essential IoT functionalities, including sensing, actuating, and computational processing. Our research on energy measurement, analysis, prediction and optimisation at both the IoT node and network level will enable energy-efficient applications of this innovative concept, paving the way for a more sustainable future in IoT technology.
Dr. Mohammud Junaid Bocus
Dr. Mohammud Junaid Bocus holds a B.Eng. (First-Class Honours) in Electronic and Communication Engineering from the University of Mauritius (2012), as well as an M.Sc. (Distinction) in Wireless Communications and Signal Processing (2014) and Ph.D. in Electrical and Electronic Engineering (2019) from the University of Bristol. His research covers wireless communication, signal processing, computer vision, and machine learning. He has contributed to EPSRC-funded projects like OPERA and NGCDI, working on passive human activity recognition, localization, joint communications and sensing, and deep learning for emergent communications. Currently, he is a senior researcher on the EU-funded SUPERIOT project at the University of Bristol, focusing on energy analysis, modelling, and optimization for sustainable IoT networks.
Mr Senhui Qiu
Senhui Qiu received his B.Sc. in Physics in 2010 and his M.Sc. in Circuits and Systems in 2013 in China. He lectured at university for eight years, leading research in deep learning and embedded systems, and also worked as a UAV engineer at Hohem Technology in China. He is completing his PhD in resource-efficient machine learning at Ulster University, UK. Currently, he is a Research Associate at the University of Bristol’s School of Computer Science, focusing on energy modelling. His expertise spans machine learning, IoT, embedded systems, and drone technology.
19th February 2025, Neural Model Checking
We introduce a machine learning approach to model checking temporal logic, with application to formal hardware verification. Model checking answers the question of whether every execution of a given system satisfies a desired temporal logic specification. Unlike testing, model checking provides formal guarantees. Its application is expected standard in silicon design and the EDA industry has invested decades into the development of performant symbolic model checking algorithms. Our new approach combines machine learning and symbolic reasoning by using neural networks as formal proof certificates for linear temporal logic. We train our neural certificates from randomly generated executions of the system and we then symbolically check their validity using satisfiability solving which, upon the affirmative answer, establishes that the system provably satisfies the specification. We leverage the expressive power of neural networks to represent proof certificates as well as the fact that checking a certificate is much simpler than finding one. As a result, our machine learning procedure for model checking is entirely unsupervised, formally sound, and practically effective. We experimentally demonstrate that our method outperforms the state-of-the-art academic and commercial model checkers on a set of standard hardware designs written in SystemVerilog.
Mr. Abhinandan Pal
Originally from Chinsurah, West Bengal, India, I'm currently pursuing my PhD in the Theory of Computer Science Group at the University of Birmingham under the supervision of Mirco Giacobbe with Daniel Kroening as an external supervisor. Prior to this, I completed my undergraduate studies at the Indian Institute of Information Technology Kalyani, where I received the President of India Gold Medal and the department's first prize. During this period, I had the privilege of engaging in research at École Normale Supérieure Ulm, Università degli Studi di Padova, and École Normale Supérieure Paris Saclay, supervised by Caterina Urban, Francesco Ranzato, Marco Zanella, and Mihaela Sighireanu, primarily focusing on the verification of machine learning models.
12th February 2025, Executable Explanations of Control Software
Cyber physical systems (CPS) play an important role in today's world. The complexity and heterogeneity of such digitally controlled systems is usually too high even for technical experts to understand the whole system in detail. One way of improving comprehensibility of such systems is providing explanations. The idea of CAUSE (Concepts and Algorithms for--and Usage of--Self-Explaining Systems) is to enable systems to explain themselves. Controllers are an essential component of CPS. A characteristic of controllers is the feedback-loop through which the output depends non-trivially on the inputs. To explain the control software, we piecewise approximate the control behaviour by simple (linear) functions. The controller is then explained in terms of these “control patterns” in an online setting where control patterns can be swapped out when outdated. The talk motivates the abstract explanation pattern introduced by CAUSE. It illustrates the concept of explanations for control software, where explanations are executable networks of timed automata, and presents first research results on online piecewise approximation of control outputs.
Ulrike Engeln received her Master's Degree from Hamburg University of Technology (TUHH) in 2023 through a dual study program with a company in the medical technology domain. She pursued working in the medical domain as engineer in innovation and technology with focus on development of new control concepts. At the same time, she joined the Institute for Software Systems where she became researcher in the research training group CAUSE in 2024. Her current research interest are executable explanations of control software.
29th January 2025, Can AVs be careful and competent?
Following four years of development and review by the Law Commissions, the Automated Vehicles Act 2024 gained royal assent setting out some of the requirements that will enable commercial exploitation of self-driving vehicles. The presentation will take us through some of the challenges in making sure that AVs meet the standards set out in the act and some of the further work that will be necessary to ensure that they gain societal acceptance.
Dr. Nick Reed
For more than twenty years, Dr Nick Reed has worked consistently at the cutting edge of transport research. In 2019, he founded Reed Mobility – an independent expert consultancy on future mobility working across public, private and academic sectors to deliver transport systems that are safe, clean, efficient, ethical and equitable and including projects for the European Commission, DfT, TfL, BSI and RSSB. Having led large scale trials of automated vehicles, much of his recent work has focused on the safety, ethics and societal implications of this technology. November 2021, he was recruited to a three-year part-time role as National Highways' first ever Chief Road Safety Adviser, developing their strategy for eliminating death and serious injury on the strategic road network.
22nd January 2025, Autonomous Aircraft Systems: Design, V&V, Certification
Unmanned Aerial Vehicles (UAVs) with advanced autonomous capabilities are gaining importance in many areas. The use of AI/ML in such safety-relevant applications poses considerable design, verification, and validation challenges.
In this talk, I will present the "Autonomous Operating System" (AOS), an autonomous flight software for UAVs developed at NASA Ames, give an overview of some relevant V&V tools developed at NASA Ames, and discuss approaches toward certification.
Dr. Johann Schumann
Dr. Johann Schumann is a KBR Senior Tech Fellow working at the NASA Ames Research Center. He received his PhD (1991) and German habilitation (2000) degrees in Computer Science from the Technische University Munich in Germany. His research work focuses on safety-critical aerospace software. Dr. Schumann is engaged in research on autonomous flight software and decision making, certification approaches for machine-learning and AI-based software systems, run-time assurance, prognostics, and the automatic generation of reliable code. He is author of a book on theorem proving in software engineering and has published more than 140 articles in relevant fields.
15th January 2025, Representation Engineering for Editing the Brains of LLMs: Detecting and Mitigating Harmful Behaviour
As the popularity of AI rises, so do methods aimed at evaluating and preventing the deployment risks it poses. However, most of those treat the network as a black box, with no insight into what is happening in the internal brain of the model. This is problematic because it does not block or quantify the random (non-deterministic) component of AI reasoning. State-of-the art techniques like representation engineering or activation patching seek to mitigate that.
Mr Lukasz Bartoszcze
Lukasz is researcher at the Alan Turing Institute and currently a third-year Feuer Scholar PhD student studying machine learning robustness, following from a successful Masters at Columbia University. Lukasz has worked on frontier model safeguards, being a contributor to LLM Guard, a popular open-source guardrail library, and experience working on hallucination mitigation techniques, automated jailbreaking and fine-tuning of models for improved robustness, as well as work on ControlAI, a unified platform for connecting regulatory requirements to specific LLM guardrails used to build auditable security evidence for AI systems. He also has worked on AI threats which includes building taxonomies of machine-learning robustness, defining appropriate threat models, and performing red-teaming, and blue-teaming and implementing safeguards directly for commercial clients against both test-time and training-time attacks during work for Palantir, BCG and Accenture in various consulting and engineering roles. Lukasz has also researched attack strategies such as malicious fine-tuning with harmful results, backdoor attacks, multilingual attacks, and ciphers against LLMs. Lukasz has worked across a range of industries, completing projects for both commercial and governmental clients in healthcare, supply chain and national defence in the UK, US, Poland and Ukraine and is also a member of ML Commons (an organisation dedicated to AI Security) and was recently a part of the UN AI security report for OSET (Office of Secretary General’s Envoy on Technology).
8th January 2025, Trustworthy Automated Driving through Qualitative Explainable Graphs
Understanding driving scenes and communicating automated vehicle decisions are key requirements for trustworthy automated driving in connected, cooperative automated mobility (CCAM). In this talk, Helge will present the Qualitative Explainable Graph (QXG), which is a unified symbolic and qualitative representation for scene understanding in urban mobility. The QXG enables interpreting an automated vehicle's environment using sensor data and machine learning models. It utilises spatio-temporal graphs and qualitative constraints to extract scene semantics from raw sensor inputs, such as LiDAR and camera data, offering an interpretable scene model. A QXG can be incrementally constructed in real-time, making it a versatile tool for in-vehicle explanations across various sensor types. Experiments have shown that 1) QXGs can be used as an action explanation mechanism, i.e. highlighting which object interactions caused actions taken by other participants; 2) Scene understanding can be strengthened when QXGs are augmented with human-labelled information about objects and relations relevance. This leads to a powerful explanation technique and fully interpretable due to the end-to-end reliance on symbolic representations and inherently interpretable machine learning techniques such as decision trees.
Dr. Helge Spieker
Helge Spieker is a Research Scientist in the Department of Validation Intelligence for Autonomous Software Systems at Simula Research Laboratory, Oslo, Norway. He received his Ph.D. from the University of Oslo in 2020. His current research interests are focused around how we can test the trustworthiness of AI systems and how both symbolic and data-driven AI can improve automated software testing.
11th December 2024, Runtime Repair for Assumption Violations in High-level Robot Controllers
Recent advancements in robotics have enabled robots to perform sophisticated navigation and manipulation skills. To fully leverage these capabilities, it is essential to design high-level controllers that can safely compose these skills to accomplish complex tasks. However, creating such controllers with correctness guarantees is a significant challenge due to the complexity of the tasks and the unpredictability of the real-world environments. Formal synthesis offers a promising solution by automatically transforming high-level specifications into correct-by-construction controllers. Yet, the synthesized controllers rely on assumptions about the environment behavior. In real-world applications, these assumptions may be violated during runtime, leading to a loss of correctness guarantees and potentially unsafe or undesired robot behaviors.
Mr Qian Meng
Qian Meng is a Ph.D. student in Computer Science at Cornell University under the guidance of Prof. Hadas Kress-Gazit. He received his B.S. in Computer Science and Mathematics from the University of North Carolina at Chapel Hill. His research focuses on applying formal methods to robotic systems to enhance their robustness and adaptability.
27th November 2024, AI in Radiology: Governance Landscape
In this talk Amrita will be discussing her keen interest in Artificial Intelligence and her efforts to set up trust wide research collaboration in the NHS to implement a digital enabled AI infrastructure. She will discuss the challenges associated with setting up and implementing novel AI software for improved detection of lung and breast cancer. Amrita aims to have a positive social impact on cancer screening within the NHS integrated with AI, working in conjunction with various stakeholders with a mission statement of patient and value-focused healthcare.
Dr. Amrita Kumar
Amrita has been recently named 2023 Top 60 Influential Women in UK for leading innovation in the use of AI within the NHS. She was appointed as a substantive Consultant Radiologist at Frimley Health in 2013 with a subspecialist interest in breast cancer screening. She has also been appointed Chair at the British Institute of Radiology National AI & Innovation Committee to look at the national integration, implementation and governance of AI into clinical practice. She is also an advisor to the AI committee at the Royal College of Radiologists.
20th November 2024,
Who is responsible for that?” A scrutiny of the ethics and trust within the human-robot paradigm in telerobotics applications
The integration of human-robot interaction, specifically robotic teleoperation, in various sectors raises significant ethical and trust-related concerns. This talk delves into the complexities of responsibility within the human-robot paradigm , particularly in telerobotics applications, with a focus on three <problem, solution> pairs: (i) enhancing telesurgery through novel surgeon-robot interfaces; (ii) alleviating firefighter workload through immersive telerobotics; and (iii) improving construction safety using novel training systems and robotic mechanisms. By analysing case studies and practical frameworks, the talk aims to provide an insight into the hows, whys, and where tos for some of the ethical considerations and trust issues towards ensuring responsible deployment of telerobotics technology.
Dr. Nikhil Deshpande
Nikhil Deshpande is an Associate Professor of Robotics and AI, with his primary affiliation with the Cyber-physical Health and Assistive Robotics Technologies (CHART) group. With over 15 years of experience in Robotics and AI covering different aspects, including navigation, manipulation, mechanism design, machine learning, computer vision, and mixed reality, he has focused on applications in telesurgery, telerobotics, therapy, industrial training, among others. Prior to joining CS @ Nottingham, Nikhil was a Researcher and Team Leader at the Italian Institute of Technology (IIT), Genova, Italy, leading the VICARIOS Mixed Reality and Simulations Lab, where his group focused on immersive remote telerobotics in hazardous environments, covering topics including remote telemanipulation, shared autonomy, 3D semantic scene understanding, haptics, mixed reality for industrial training, control simulations, etc. Nikhil has been the lead organiser of the XR-ROB workshop, the only gathering of its kind at the IEEE/RSJ IROS international conference exploring the confluence of extended reality (XR) and robotics technologies. Nikhil has been PI and co-I for multiple collaborative projects, with R&D funding exceeding €7 million, working closely with stakeholders, including surgeons, firefighters, and construction workers, and demonstrated the project outcomes in public dissemination events, including to the President of Italy in 2022. Nikhil received his PhD in Electrical Engineering, with a focus on Robotics, in 2012, from the North Carolina State University (NCSU), USA, Master's in Integrated Manufacturing Systems Engineering at NCSU in 2007, and his Bachelor's in Electrical Engineering in 2003 from the College of Engineering (COEP), Pune, India.
13th November 2024, Assessing quality and reliability of robotic software for industrial applications ...
The global supply chains face emerging and ever growing challenges across industries, highlighted during the COVID-19 pandemic and diverse political events over the last couple of years. Supply chain disruption has become more dynamic, and the requirement for dealing with a high mix of products through many distribution channels has increased with the demand for mass customization. The need for agile and flexible robot solutions controlled by intricate software (e.g. Robot Operating System) with the latest artificial intelligence advancements, modular software architectures, easy to use interfaces and adaptability capabilities has become more apparent as means to drive business value and increase resiliency. As robots become smarter and take over more complex tasks, the challenges to ensure their safety, cybersecurity and reliability will increase. In this talk, we will take note of current and future technological trends in robotic software for supply chain applications, new and increasing challenges at assessing quality and reliability of robotic software solutions applied to manufacturing and logistics, and briefly reflect on possible ways to address such challenges through software engineering and verification and validation tools and techniques, to open room for thought and collaboration.
Dr. Dejanira Araiza-Illan
Dr. Dejanira Araiza-Illan is an assistant principal engineer in Robotic Applications at the Enterprise Supply Chain Advanced Technology team, at Johnson & Johnson. Alongside, she is currently doing a stretch assignment on automation and digital innovation at the company's clinical supply chain. Her professional interests include industrial advanced robotic applications, software engineering for robotics, and verification and validation of autonomous systems. She is also a co-chair of the IEEE Robotics and Automation Technical Committee on the Verification of Autonomous Systems. Previously, she worked as a scientist and software developer at the ROS-Industrial Consortium Asia Pacific and the Advanced Remanufacturing and Technology Centre at A*STAR, in Singapore. She also contributed to the UK funded projects RIVERAS and ROBOSAFE, on the verification of control systems and trustworthy robotic assistants, as a postdoctoral researcher in the University of Bristol. She holds a PhD in Automatic Control and Systems Engineering by the University of Sheffield, UK.
6th November 2024, Forward and Backward Analysis for Neural Network Certification
In the past decade, artificial intelligence (AI), especially deep learning (DL), has achieved significant advancement. Despite the wide deployment and enthusiastic embrace of AI technologies, the instability and black-box nature of DL systems are raising concerns about the readiness and maturity of AI. As with any automation technology, certification is an essential step to take for AI to be deployed in real-world safety- and security-critical applications. In this talk, I will present recent research outcomes for forward and backward analysis of neuron networks (NNs) to provide provable guarantees on the critical decisions made by NN-based systems. We propose an automated convex bounding algorithm for forward analysis of neural networks with general activation functions. For backward analysis, we present an efficient anytime algorithm to derive preimage approximations, which enables sound and complete quantitative verification for piecewise-linear neural networks.
Dr. Xiyue Zhang
Xiyue Zhang is a Lecturer in the School of Computer Science at the University of Bristol. Before joining Bristol, she was a Research Associate in the Department of Computer Science at the University of Oxford. She received her PhD in 2022 from Peking University, where she focused on trustworthiness assurance of deep learning systems, and her BSc in 2017, also from Peking University. Her research mainly focuses on trustworthy deep learning, integrating both provable certification and practical empirical methods. Her recent work includes abstraction and verification for deep learning models and deep learning-enabled systems.
16th April 2025, Finding and Quantifying Rare Safety Violations in Autonomous Vehicle Simulations
Virtual scenarios allow developers to rapidly test vehicles in safety-critical conditions before considering costly real road tests. However, even with sophisticated physical simulation, such scenarios contain myriad sources of randomness from sensor noise, perception, and traffic behaviour. One might run 1000 simulations and get 1000 differing outcomes. Worse, high-risk outcomes (like collisions) can be extremely rare. All of this is exacerbated by the prevalence of "black box" AI components within vehicle stacks, which make systems less amenable to traditional engineering analyses.
To answer the simple question of "How likely is it that my vehicle will violate this safety property?", I will present our work on adaptive sampling and risk-driven design. By leveraging machine learning techniques to learn simulation factors that are more likely to result in safety violations, our methods produce more simulations in critical failure regions, and thus get a more accurate picture of the problem areas of a given autonomous vehicle system.
Dr. Craig Innes
Craig Innes is a Chancellor's Fellow Lecturer at the University of Edinburgh within the Institute for Perception, Action, and Behaviour. He has served as a Co-Investigator on the UKRI Trustworthy Autonomous Systems Hub on Computational Tooling, and has worked as part of multiple projects around the themes of Machine Learning and Cyber-Physical Safety.